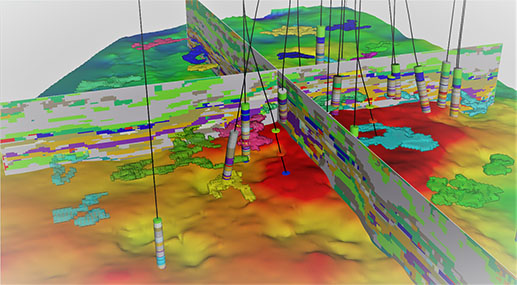
Rock type identification is an important process developed in reservoir characterization studies that help to identify and estimate different petrophysical and geological parameters to describe the storage capacity and transmissibility characteristics in porous media. This assessment is particularly useful at the time of studying and assigning rock properties along different geological units. After, these characteristics are established, it is simple to classify geological units that share similar characteristics.
The methods of rock image recognition mainly include gravity and magnetism, well logging, seismic, remote sensing, electromagnetic, geochemistry, hand specimen, thin section analysis, etc. The traditional rock image recognition and classification is mainly based on naked-eye observation, which has long periods, difficult quantification and low recognizable efficiency.
Machine learning algorithms can be used to overcome this sort of limitation. These kind of algorithms make it possible to classify large amounts of observations in certain numbers of classes with a previously known pattern, where each class is comprised of observations that share similar attributes, but different compared with observations from other classes. Machine learning algorithms have been applied effectively in many other Oil and Gas disciplines such as geophysics, petrophysics, reservoir and production engineering.
The outcome of this process is a probabilistic facies model description of the reservoir. It predicts the most probable facies distribution and associated maximum probability, and the probability relative to each facies. This results in less guesswork when quantifying uncertainty in rock type distribution. The outcome is critical for reservoir geologists and engineers to better understand reservoir behavior.
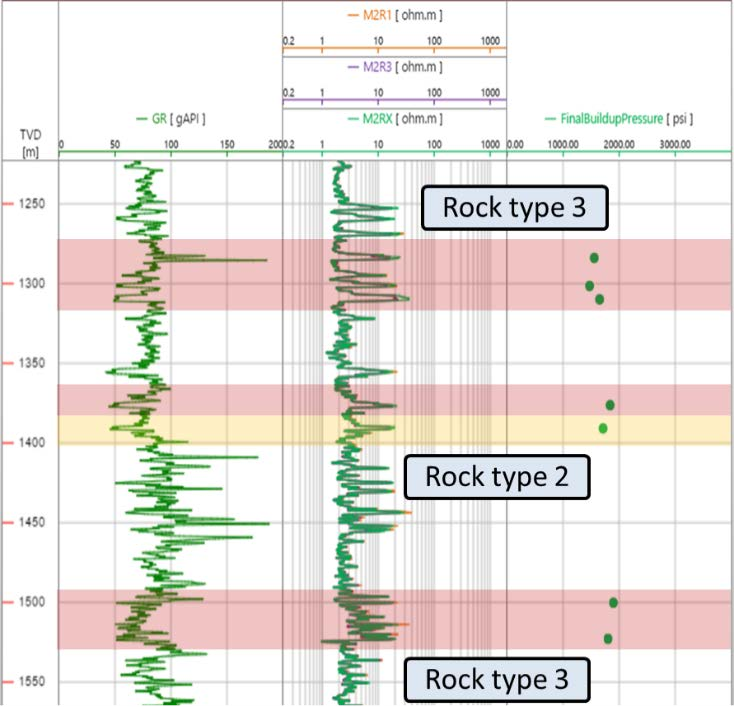
Once considered “nice-to-have technologies”, the sheer volume of well and seismic data that needs to be analyzed has made Machine Learning an effective approach for transformation and analysis of subsurface data. Automated Machine Learning produces outputs in minutes or hours rather than months or years. And, since Machine Learning integrates data of different resolutions (core, wireline and seismic data) and different domains, it becomes a collaboration agent where geologists, reservoir engineers, and geophysicists can work together to ensure that disparate data is calibrated, and results validated.