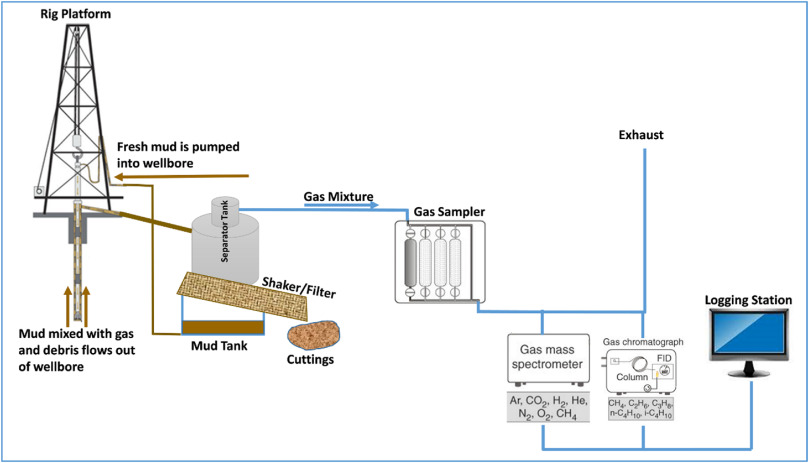
Mud data is acquired during the drilling of oil and gas wells. It is part of the mud logging process which characterizes the liquid and solid components of the debris that are brought to the surface along with mud. With respect to the liquid component of mud, the mud logging process involves the quantitative measurement of the gas liberated from the subsurface formations as the drill bit grinds through rock formations. With respect to the solid component, the mud logging process includes the analysis of the rock fragments (drill cuttings). For the former, hydrocarbon gas detectors are used to measure the individual natural gas components dissolved in the mud and brought up to the surface through mud circulation. Gas chromatographs and mass spectrometers are used to determine the chemical composition of the gases for adequate separation and quantification. The main objective of measuring liberated gas is to identify all hydrocarbon indications from the formation as they are trapped in the drilling mud for possible indication of drilling anomalies, reservoir properties, and hydrocarbon shows.
In the past, the traditional utilization of mud gas data has happened in three major ways:
- correlation with geological or petrophysical information
- formation evaluation
- formation fluid typing
These three applications of mud data have been interesting and beneficial to the petroleum geology field despite the various issues around the quality of data. Can we do more with this data? more precisely:
- With the recent shift from qualitative to quantitative reservoir characterization, could the utility of mud data be extended to this advantage?
- With the digital transformation effort of the petroleum industry, could mud data be utilized for real-time reservoir characterization?
One key component of the fourth industrial revolution and an enabler of the digital transformation process is the application of machine learning (ML). ML seeks to teach computers to do what humans naturally do without being explicitly programmed. Through ML, algorithms are able to continuously learn from patterns embedded in historical data and adaptively improve their predictive capabilities as more data is added.
Using mud data together with ML algorithms can result in the following applications:
- Predict fluid types in real-time
- Predict gas oil ratio in real-time
- Identify deep coal seam family
- Predict reservoir fluid properties
- Predict gas kick incidents
- Predict reservoir porosity
Also, smart fusion of mud data with other data types (wireline logs, geological, geochemical, drilling logs, gamma ray, …) can lead to new profitable avenues in the oil & gas industry. Some of these applications include:
- Detect influx and mud loss
- Detect drilling events
- Predict Equivalent Circulating Density (ECD)
- Detect nonproductive times while drilling
- Predict and optimize ROP
- Classify drilling status
- Provide early warning system for stuck pipe
- Detect lost circulation
- Predict lithology