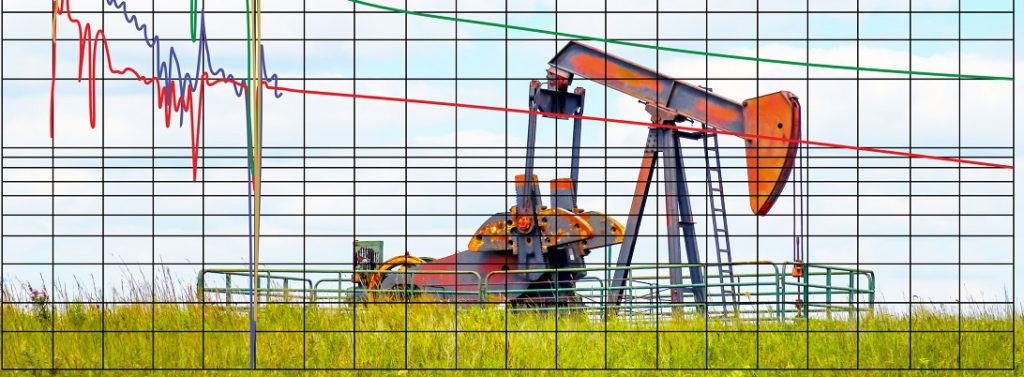
The technological advancement and competitive environment have changed the entire dynamics of modern businesses. To compete and grow with modern businesses and workflows, many areas such as healthcare, engineering, and heavy mechanical industries are trying to implement solutions that are highly effective, precise, and less time taking. On the other hand, Oil and Gas Industry especially Exploration and Production Department has been slower than other industries in realizing the need for change/advancement. With the complexities in the processes and heterogeneity of the reservoirs, there is a need for a more intelligent system that can consider all the aspects of understanding downhole complexities and surface control systems to optimize the systems and improvise the decision making.
Understanding Reservoir Behavior has always been a challenge because of many complexities such as fluid behavior with changing downhole pressure, the effect of production rate on the reservoir, and depletion of the fields. Reservoir Modelling has faced many challenges and there is a need of using machine learning and intelligent solutions to improvise the understanding of Reservoir and enhancing the accuracy of Reservoir Models. In the process of field development and production optimization, well placement, estimation of oil-water and gas-oil contacts have been a recurring problem. Many industrial simulators have been limited in their capacity to consider geological complexities, spatial data, production data, and rock properties for a single model, and therefore, the results from those simulators have been badly compromised.
Understanding Reservoir Behavior using Machine Learning
Time and Pressure are the two factors that continuously affect the reservoir behavior by changing the fluid properties and petrophysical properties of the rock. This change is an important aspect in estimating reservoir performance and management. Reservoir Response and Performance can be estimated accurately using numerical simulations but these numerical simulators somehow limit the applications and extent of estimation because of multi-dimensional changes in the parameters such as production rates, pressures, saturations, and fluid properties. To overcome this limitation, machine learning and artificial intelligence are being implemented.
Implementation of Machine Learning using Fast Marching Methods, Gradient Boosting Methods, LSTM, and RNN can help in understanding the reservoir behavior in terms of pressures, performance prediction of the production fields on the basis of producing rates, and reservoir properties. Machine Learning can also be helpful in predicting the reservoir properties outside the dataset and analyze the petro-physical properties of the reservoir. With the help of Machine Learning, geological modeling of the reservoirs using the continuous variable modeling method can provide accurate results.
Applications
When understanding the reservoir behavior, we have to deal with huge data such as seismic data, well logs, geological data, drilling, and production history. To extract the important information and parameters for analysis, we have to go through each bit of data. Machine Learning makes it easy to extract the data from bundles of information and pick the necessary information to analyze. Data automation can be done to collect, extract and sort the data into the desired format. Artificial Neural Networks and other models are being used to estimate downhole pressures and reservoir productivity & recovery factor. Complex fault analysis and geological modeling of the reservoirs have been done to predict the formation behavior and stratigraphy prior to drilling a well. With the help of Machine Learning, a combination of NMR logs with production data has provided insight into fluid composition and pore size distribution of the rock. A combination of Well logs data, Seismic data, and production history can be used to model production forecasting to get infill drilling locations, well performance assessment, production sweep efficiency. Similarly, the combination of numerical simulators and AI models can help in performing Monte Carlo Simulations, Reserve Optimization, and EOR modeling.
As a summary, the following are methods used in Oil & Gas Industry:
- Gradient Boost Method provides Accurate Well Placement
- Hyper-Dimensional Procedure for Well Placement and Control Analysis
- Reservoir Modelling can be done with Connectivity-Aided ML
- Well Performance Forecasting can be down with LSTM
- Geological Modelling can be done with BNN and hybridized geo-statistical ML (Nwachukwu, Jeong, Pyrcz, & Lake, 2018)
Conclusion
In past, there was a big challenge of handling huge data volume for reservoir management and field development for future wells. Engineers were making many assumptions at the initial level of reservoir modeling and then it was difficult to change already developed models with the updated data. With the advancement in Machine Learning and Neural Networks, it has become easier to develop models on the basis of big data volume and then extracting the required information, sort and analyzing it for future predictions. In a nutshell, Machine Learning has helped us understand reservoir behavior better and easier than ever.
- Using ML, 3D seismic data can be interpreted automatically and fault extraction can be done
- Real-time production optimization can be done using artificial intelligence
- Business based models can be developed using Machine Learning
- Accurate reservoir parameters can be estimated using combined data analysis
- Future reservoir performance can be predicted with recurring neural networks and time-series forecasting
- Primary Recovery data, injection wells data, and production history can be utilized to model enhanced and advanced oil recovery projects
- Screening criteria of selecting the Artificial Lift Techniques and Enhanced Oil Recovery Techniques can be automated using Machine Learning
- Sensitivity Analysis, History Matching, and Well Test Analysis curve matching can be done with ML instead of using multiple simulations runs.